Artificial Intelligence (AI) fuels innovation and has transformed industries, from healthcare to finance and fashion, and everything in between However, as AI expands as a tool with more use cases and applications, the environmental impact of this revolutionary technology is simultaneously driving increasing concerns.
AI has the potential to truly usher in a new age of understanding and prosper, unlocking mysteries like dark energy and helping solve huge problems such as climate change (for example, OWL’s advanced AI provides reliable and timely data to make sustainable investment decisions aligned with ESG principles). But AI comes with significant environmental impact as it stands, given its huge energy demands and large servers and databases, including increases in carbon emissions, electronic waste, and potential harm to ecosystems.
AI’s carbon footprint and ESG data
The carbon footprint associated with AI technology spans across its development, maintenance, and disposal phases. As datasets and models grow in complexity, the energy requirements for training and operating AI models become increasingly substantial, directly contributing to higher greenhouse gas emissions (GHG) and exacerbating climate change.
OpenAI researchers highlight a concerning trend, noting that the computing power needed to train state-of-the-art AI models has doubled approximately every 3.4 months since 2012. By 2040, the Information and Communications Technology (ICT) industry is projected to contribute 14 percent of global emissions, with a significant share stemming from ICT infrastructure, specifically data centers and communication networks. These findings underscore the pressing need to address AI’s environmental impact and its role in accelerating environmental degradation.
A 2019 study conducted by researchers at the University of Massachusetts delved into the energy consumption of popular large AI models during the training process. The results revealed that training alone can generate around 626,000 pounds of carbon dioxide—equivalent to roughly 300 round-trip flights between New York and San Francisco, nearly five times the lifetime emissions of an average car.
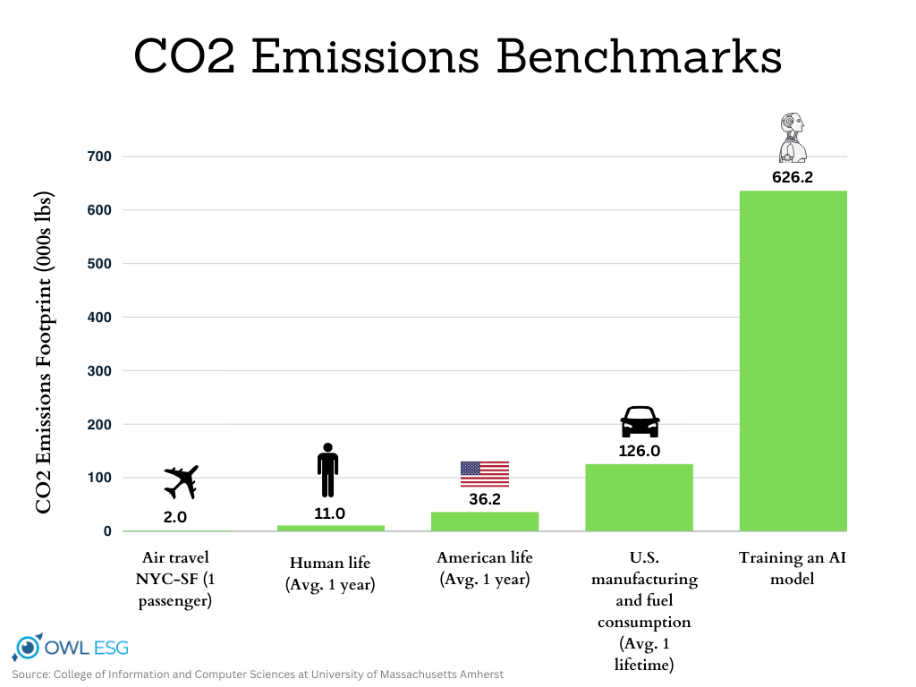
Electronic waste disposal
AI technology contributes significantly to electronic waste (e-waste), which contains hazardous chemicals like lead, mercury, and cadmium. These substances pose threats to soil, water supplies, and overall ecological well-being, endangering both human health and the environment.
Effectively managing e-waste and recycling are essential measures for alleviating environmental harm and reducing the release of harmful compounds. To responsibly process and recycle AI-generated electronic waste (reducing its environmental impact) stricter regulations are coming. Additionally, ethical disposal practices are crucial.
Research studies have shown that AI can assist in e-waste collection planning. For instance, machine learning models trained by AI algorithms can enhance supply chain and recycling efficiently by improving inventory levels and identifying, collecting, and sorting various components from electronic devices and other forms of waste.
Impact of Natural Ecosystems
AI applications such as self-driving cars and delivery drones pose a risk to wildlife and ecosystems. AI-driven automation, especially in fast-paced industries like e-commerce, may increase consumption and waste.
For instance, there are currently about 50 million tons of e-waste generated annually, surpassing the combined weight of all commercial airplanes ever manufactured. If nothing changes, it’s estimated that the yearly amount of e-waste could more than double by 2050.
And, out of all electronics, roughly 20 percent is recycled through organized and regulated channels, with most ending up in landfills. Improper disposal of e-waste in landfills leads to soil and groundwater contamination. More important, informal handling of e-waste poses significant health risks to workers and communities due to the presence of hazardous components like mercury, lead, bromine, and arsenic in electronic devices. For instance, prolonged exposure to arsenic, common in microchips, can result in lung cancer, nerve damage, and skin diseases, while lead exposure can lead to brain and kidney damage, blood disorders, especially in children.
The expanding use of AI in agriculture also raises concerns about excessive pesticide and fertilizer use, resulting in soil and water contamination and harm to biodiversity. Implementing AI to enhance agricultural practices, potentially at the cost of ecosystem health, could lead to the development of monocultures and biodiversity decline.
Ethical concerns regarding AI in environmental management are rising due to potential biases from incomplete or inaccurate data. For example, prioritizing economic growth over environmental protection may lead to short-term financial gains at the expense of sustainability.
Regulations
Since 1992, the Basel Convention on the Control of Transboundary Movements of Hazardous Wastes and Their Disposal has prohibited the export of e-waste from developed to developing countries. And in 1995, parties to the Basel Convention adopted the Ban on Exporting Hazardous Waste to Developing Countries, extending the prohibition to members of the EU, Organization for Economic Co-operation and Development (OECD), and Liechtenstein. This Ban became effective on December 5, 2019, with 98 ratified countries.
The UN is also addressing the increasing problem of e-waste. Several agencies such as the International Telecommunications Union (ITU), International Labour Organization (ILO), and the UN Environment Programme (UNEP), have highlighted why it’s crucial we confront the global e-waste challenge in a timely manner.
Following suit, in March 2018, these agencies (among others) signed a ‘Letter of Intent’ to establish coordination and collaboration mechanism addressing e-waste. This initiative led to the formation of the E-Waste Coalition, which advocates for knowledge-sharing, best practices, and the development of a unified intervention model for national e-waste policies.
2023 was the boom for AI policy and regulation. In the U.S., AI entered the political conversation, with President Biden’s executive order on AI released at the end of October. The order mandates increased transparency from AI companies regarding their model operations and introduces new standards, notably for labeling AI-generated content. Aimed at enhancing “AI safety and security,” the White House emphasized sharing safety test results for new AI models with the U.S. government, calling upon the Defense Production Act to address potential national security risks—an unexpected measure typically reserved for times of national emergency.
For more information on the state of e-waste laws and regulations worldwide, check out The Global E-Waste Monitor.
However, some AI researchers say this approach is a cause for concern because it ignores how timely it is to train and develop models to decrease foreseeable harm(s). Rather than focusing on preemptive measures to prevent AI-related harms before deployment, the White House is adopting a “whack-a-mole” approach by addressing issues that have already surfaced.
Ensuring the sustainable future of AI
Through the optimization of both hardware and algorithms, AI systems can reach comparable efficiency while using less energy. Fostering transparency and accountability is also crucial. Stakeholders need to prioritize providing relevant data on AI models and sources for assessing environmental impact.
Governments and regulatory agencies should implement specific standards and restrictions to ensure ethical AI development, utilization, and disposal. Collaborative efforts among businesses, academics, and policymakers are also essential and should focus on solutions that combine technological advancements with environmental sustainability through multidisciplinary research.